layout: true .hheader[<a href="index.html"><svg style="height:0.8em;top:.04em;position:relative;fill:steelblue;" viewBox="0 0 576 512"><path d="M488 312.7V456c0 13.3-10.7 24-24 24H348c-6.6 0-12-5.4-12-12V356c0-6.6-5.4-12-12-12h-72c-6.6 0-12 5.4-12 12v112c0 6.6-5.4 12-12 12H112c-13.3 0-24-10.7-24-24V312.7c0-3.6 1.6-7 4.4-9.3l188-154.8c4.4-3.6 10.8-3.6 15.3 0l188 154.8c2.7 2.3 4.3 5.7 4.3 9.3zm83.6-60.9L488 182.9V44.4c0-6.6-5.4-12-12-12h-56c-6.6 0-12 5.4-12 12V117l-89.5-73.7c-17.7-14.6-43.3-14.6-61 0L4.4 251.8c-5.1 4.2-5.8 11.8-1.6 16.9l25.5 31c4.2 5.1 11.8 5.8 16.9 1.6l235.2-193.7c4.4-3.6 10.8-3.6 15.3 0l235.2 193.7c5.1 4.2 12.7 3.5 16.9-1.6l25.5-31c4.2-5.2 3.4-12.7-1.7-16.9z"/></svg></a>] --- class: center, middle, inverse # Forecasting Time Series ## Time-varying Regression .futnote[Eli Holmes, UW SAFS] .citation[eeholmes@uw.edu] --- ## Time-varying regression Time-varying regression is simply a linear regression where time is the explanatory variable: `$$log(catch) = \alpha + \beta t + \beta_2 t^2 + \dots + e_t$$` The error term ( `\(e_t\)` ) was treated as an independent Normal error ( `\(\sim N(0, \sigma)\)` ) in Stergiou and Christou (1996). If that is not a reasonable assumption, then it is simple to fit an autocorrelated error model or non-Gausian error model in R. --- Stergiou and Christou (1996) fit time-varying regressions to the 1964-1987 data and show the results in Table 4. 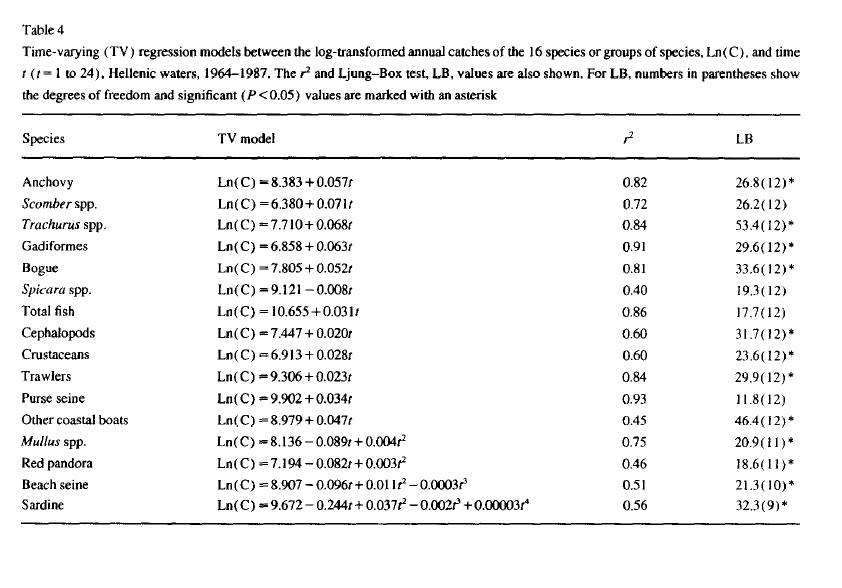 --- The first step is to determine how many polynomials of `\(t\)` to include in your model. <img src="Forecasting_2_-_TV_Regression_files/figure-html/poly.plot-1.png" style="display: block; margin: auto;" /> --- Here is how to fit a linear regression to the anchovy landings with a 4th-order polynomial for time. We are fitting this model: `$$log(Anchovy) = \alpha + \beta t + \beta_2 t^2 + \beta_3 t^3 + \beta_4 t^4 + e_t$$` ```r landings$t = landings$Year-1963 model <- lm(log.metric.tons ~ poly(t,4), data=landings, subset=Species=="Anchovy"&Year<=1987) ``` --- They do not say how they choose the polynomial order to include. We will look at the fit and keep the significant polynomials. ```r summary(model) ``` ``` ## ## Call: ## lm(formula = log.metric.tons ~ poly(t, 4), data = landings, subset = Species == ## "Anchovy" & Year <= 1987) ## ## Residuals: ## Min 1Q Median 3Q Max ## -0.26951 -0.09922 -0.01018 0.11777 0.20006 ## ## Coefficients: ## Estimate Std. Error t value Pr(>|t|) ## (Intercept) 9.17747 0.03541 259.213 < 2e-16 *** ## poly(t, 4)1 6.06211 0.55180 10.986 1.13e-09 *** ## poly(t, 4)2 1.77153 0.59015 3.002 0.00733 ** ## poly(t, 4)3 0.68734 0.57016 1.206 0.24280 ## poly(t, 4)4 -0.49989 0.51403 -0.972 0.34302 ## --- ## Signif. codes: 0 '***' 0.001 '**' 0.01 '*' 0.05 '.' 0.1 ' ' 1 ## ## Residual standard error: 0.1458 on 19 degrees of freedom ## Multiple R-squared: 0.9143, Adjusted R-squared: 0.8962 ## F-statistic: 50.65 on 4 and 19 DF, p-value: 7.096e-10 ``` --- This suggests that we keep only the 1st polynomial, i.e. a linear relationship with time. ```r dat = subset(landings, Species=="Anchovy" & Year <= 1987) model <- lm(log.metric.tons ~ t, data=dat) ``` The coefficients and adjusted R2 are similar to that shown in Table 4. ```r c(coef(model), summary(model)$adj.r.squared) ``` ``` ## (Intercept) t ## 8.36143085 0.05818942 0.81856644 ``` --- We want to test if our residuals are independent. We can do this with the Ljung-Box test as Stergio and Christou (1995) do. For the Ljung-Box test * Null hypothesis is that the data are independent * Alternate hypothesis is that the data are serially correlated Example: ```r Box.test(rnorm(100), type="Ljung-Box") ``` ``` ## ## Box-Ljung test ## ## data: rnorm(100) ## X-squared = 1.3181, df = 1, p-value = 0.2509 ``` The null hypothesis is not rejected. These are not serially correlated. --- Stergio and Christou appear to use a lag of 14 for the test (this is a bit large for 24 data points). The degrees of freedom is lag minus the number of estimated parameters in the model. So for the Anchovy data, `\(df = 14 - 2\)`. ```r x <- resid(model) Box.test(x, lag = 14, type = "Ljung-Box", fitdf=2) ``` ``` ## ## Box-Ljung test ## ## data: x ## X-squared = 27.18, df = 12, p-value = 0.007279 ``` Compare to the values in the far right column in Table 4. The null hypothesis of independence is rejected. --- For the sardine (bottom row in Table 4), Stergio and Christou fit a 4th order polynomial. There are two approaches you can take to fitting n-order polynomials. The first is to use the `poly()` function. This creates orthogonal covariates for your polynomial. What does that mean? Let's say you want to fit a model with a 2nd order polynomial of `\(t\)`. It has `\(t\)` and `\(t^2\)`, but using these are highly correlated. They also have different means and different variances, which makes it hard to compare the estimated effect sizes. The `poly()` function creates covariates with mean and covariance or zero and identical variances. ```r T1 = 1:24; T2=T1^2 c(mean(T1),mean(T2),cov(T1, T2)) ``` ``` ## [1] 12.5000 204.1667 1250.0000 ``` ```r T1 = poly(T1,2)[,1]; T2=poly(T1,2)[,2] c(mean(T1),mean(T2),cov(T1, T2)) ``` ``` ## [1] 4.921826e-18 2.674139e-17 -4.949619e-20 ``` --- With `poly()`, a 4th order time-varying regression model is fit to the sardine data as: ```r dat = subset(landings, Species=="Sardine" & Year <= 1987) model <- lm(log.metric.tons ~ poly(t,4), data=dat) ``` This indicates support for the 2nd, 3rd, and 4th orders but not the 1st (linear) part. --- ```r summary(model) ``` ``` ## ## Call: ## lm(formula = log.metric.tons ~ poly(t, 4), data = dat) ## ## Residuals: ## Min 1Q Median 3Q Max ## -0.115300 -0.053090 -0.008895 0.041783 0.165885 ## ## Coefficients: ## Estimate Std. Error t value Pr(>|t|) ## (Intercept) 9.31524 0.01717 542.470 < 2e-16 *** ## poly(t, 4)1 0.08314 0.08412 0.988 0.335453 ## poly(t, 4)2 -0.18809 0.08412 -2.236 0.037559 * ## poly(t, 4)3 -0.35504 0.08412 -4.220 0.000463 *** ## poly(t, 4)4 0.25674 0.08412 3.052 0.006562 ** ## --- ## Signif. codes: 0 '***' 0.001 '**' 0.01 '*' 0.05 '.' 0.1 ' ' 1 ## ## Residual standard error: 0.08412 on 19 degrees of freedom ## Multiple R-squared: 0.6353, Adjusted R-squared: 0.5586 ## F-statistic: 8.275 on 4 and 19 DF, p-value: 0.0004846 ``` --- However, Stergiou and Christou used a raw polynomial model using `\(t\)`, `\(t^2\)`, `\(t^3\)` and `\(t^4\)` as the covariates. We can fit this model as: ```r dat = subset(landings, Species=="Sardine" & Year <= 1987) model <- lm(log.metric.tons ~ t + I(t^2) + I(t^3) + I(t^4), data=dat) ``` The coefficients and adjusted R2 are similar to that shown in Table 4. ```r c(coef(model), summary(model)$adj.r.squared) ``` ``` ## (Intercept) t I(t^2) I(t^3) I(t^4) ## 9.672783e+00 -2.443273e-01 3.738773e-02 -1.983588e-03 3.405533e-05 ## ## 5.585532e-01 ``` --- The test for autocorrelation of the residuals is ```r x <- resid(model) Box.test(x, lag = 14, type = "Ljung-Box", fitdf=5) ``` ``` ## ## Box-Ljung test ## ## data: x ## X-squared = 32.317, df = 9, p-value = 0.0001755 ``` `fitdf` specifies the number of parameters estimated by the model. In this case it is 5, intercept and 4 coefficients. The p-value is less than 0.05 indicating that the residuals are temporally correlated. --- Although Breusch-Godfrey test is more standard for regression residuals. The forecast package has the `checkresiduals()` function which will run this test and some diagnostic plots. ```r library(forecast) checkresiduals(model) ``` 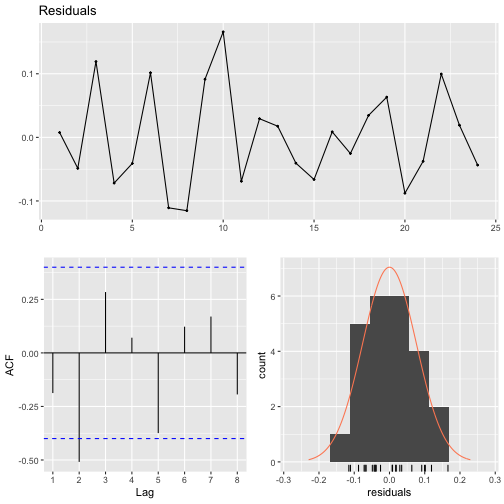<!-- --> ``` ## ## Breusch-Godfrey test for serial correlation of order up to 8 ## ## data: Residuals ## LM test = 14.6, df = 8, p-value = 0.06741 ``` --- class: center, middle, inverse # Summary --- ## Why use time-varying regression? * It looks there is a simple time relationship. If a high-order polynomial is required, that is a bad sign. * Easy and fast * Easy to explain * You are only forecasting a few years ahead * No assumptions required about 'stationarity' --- ## Why not to use time-varying regression? * Autocorrelation is not modeled. That autocorrelation may hold information for forecasting. * You are only using temporal trend for forecasting (mean level). * If you use a high-order polynomial, you might be modeling noise from a random walk. That means interpreting the temporal pattern as having information when in fact it has none. ## Is time-varying regression used? All the time. Most "trend" analyses are a variant of time-varying regression. If you fit a line to your data and report the trend or percent change, that's a time-varying regression.